When choosing a name for this note, I wanted something clickbait-ey and provocative. “Calling Bullshit on AI Hype” felt right. In recent years, Artificial Intelligence has been surrounded by immense hype. Challenging a hype cycle is often an effective way to capture attention. So, now that I have your attention, let me start by acknowledging that AI is indeed a marvel of modern technology, deserving much of its acclaim. However, it is important to approach this field with realism and level-headedness. We must move beyond the hype and recognize current limitations to harness AI’s potential for humanity’s benefit.
I want to assume we all know what Artificial Intelligence is. So let me not bore you with a definition. If you’ve been living under a rock and haven’t heard of Artificial Intelligence, here’s a link you can read up on 🙃.
First, I want to express great respect for everyone who has contributed to advancing the field of artificial intelligence. Thanks to their incredible work, we have applied AI to solve a myriad of fascinating problems:
- We have made significant strides in Natural Language Processing, enabling us to process and generate text.
- We have developed autonomous driving systems, applying AI to road navigation.
- We have advanced our understanding of biological processes and protein structure prediction, facilitating new drug discoveries.
- With large models trained across different modalities (text, audio, video), machines can now perform various tasks.
- AI has been applied to climate modeling, speech recognition, facial recognition, and many other areas.
Modern AI often relies on function fitting, training models with example (x, y) pairs to create a function f that approximates y for any x. This works well with diverse and high-quality training sets but struggles with complex problem spaces like generative AI and self-driving cars where it is impossible for training data to cover the entire problem space.
Even with extensive data, such as entire internet scrapes for Large Language Models, AI systems still perform poorly on novel tasks. Self-driving cars still make critical errors in unencountered scenarios.
Humans, in contrast, excel at reasoning and common sense.
Artificial Neural Networks (ANNs) have advanced AI significantly, enabling applications in Natural Language Processing, Speech Recognition, and more. However, ANNs based on statistical methods struggle with tasks that require pure reasoning.
Inspired by the complex and adaptable Biological Neural Networks (BNNs) found in animals, ANNs are still a simplified approximation. BNNs’ complexity and neuroplasticity allow continuous learning, highlighting the gap AI has yet to bridge. Despite progress, much work remains in developing ANNs.
What are the limitations of ANNs?
Data Inefficiency
AI systems, particularly Large Language Models (LLMs) like GPT-4 and Gemini, require enormous amounts of data. These models are trained on vast datasets scraped from the internet, including blogs, news sites, forums, and tweets. For years, Big Tech companies have collected extensive datasets to train these models. Despite their ability to store vast amounts of information, LLMs often produce factually unreliable outputs, sometimes hallucinating or struggling with tasks vastly different from their training data. To remain accurate and relevant, LLMs need continual retraining on new data.
While humans also need exposure to new information to learn, we are significantly more data-efficient. We can extract more knowledge from the same amount of data. We can draw isomorphisms to apply existing knowledge to uncharted territory. We can combine information from multiple domains to generate unique insights. Unlike AI, we don’t need to be fed data from the entire internet to understand new concepts.
Current research aims to improve machine learning systems’ efficiency by focusing on techniques like zero-shot learning, transfer learning, and more efficient model architectures. While these efforts show promise, it will take time to significantly reduce AI’s data dependency.
Energy Inefficiency
The energy costs involved in training and running Large Language Models (LLMs) are substantial. For example, training a model like GPT-4 required thousands of MegaWatts of energy. In contrast, the human brain consumes about 20 watts of energy on average. While the human brain isn’t exactly processing massive amounts of internet data, this stark contrast highlights the inefficiency of current AI models.
To meet today’s demand, significant efforts are needed to make AI systems more energy-efficient. We need advancements in hardware to improve energy efficiency, enabling more work per unit of energy (performance per watt). Specialized hardware for machine learning, such as Graphics Processing Units (GPUs) and Tensor Processing Units (TPUs), are already in use. However, considerable research is aimed at further improving the energy efficiency of these devices to achieve much better performance.
Poor at Generalizing
Generalization refers to a model’s ability to handle data or scenarios vastly different from those encountered during training. Current AI systems struggle with generalization, which is why they require vast amounts of data to cover a sufficiently large area of the problem space. This relates to the data inefficiency discussed earlier.
Humans, on the other hand, are inherently good at generalizing. We can gobble up limited information about a concept and use intuition to fill in the missing pieces as we navigate novel scenarios outside our body of knowledge. To create truly intelligent systems, we need models that are more reliable at generalizing. This necessity for better generalization leads to the next point.
Limited Reasoning
Reasoning is what it comes down to when it’s time to reliably generalize beyond training data. This goes beyond recalling something you have seen in training. Reasoning requires understanding. Cognitive abilities that currently AI systems seem to struggle with. Case in point:

How about this one from my friend Adora who tried the same prompt with Gemini:
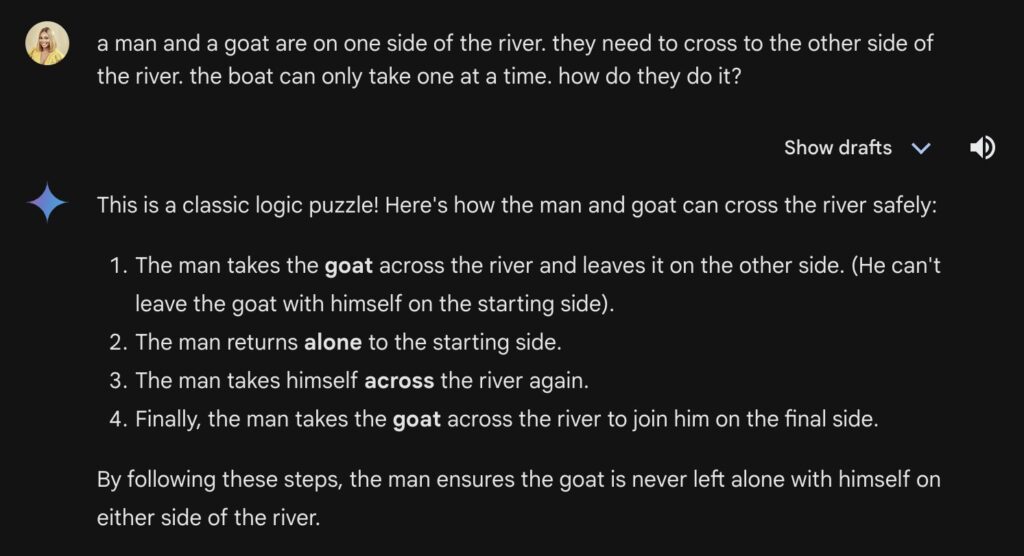
In the prompt above, we modified the classic lion, goat, and grass river crossing puzzle to make it unsolvable. ChatGPT and Gemini with full confidence spat out illogical responses. It’s easy to see how they came up with their answers. Based on training data they had memorized on the original problem, they tried to approximate an answer to our question, which was never going to work.
Reasoning still remains a big problem in Artificial Intelligence. Today’s LLMs do not carry out reasoning in the way we humans do. They are largely based on next-token prediction – which isn’t the same as reasoning in the real sense.
The breakthrough we need to unlock reasoning in machines lies at the intersection of cognitive science and mathematics. I’m taking software engineering for granted because once those other domains come together, software engineering will find a way.
Static Nature
Artificial Neural Networks (ANNs) typically have a fixed architecture with a predetermined number of nodes, connections, and layers. This static nature contrasts sharply with the dynamic, ever-evolving neural networks of the human brain, which continuously form new neural pathways. This adaptability allows humans to learn continuously and adjust to new scenarios.
One of the significant challenges in AI research is enabling continuous learning in ANNs, allowing them to update their models as they encounter new data. Instead of relying on large, static models trained on extensive datasets, future advancements should focus on developing networks that can learn incrementally and adjust their internal parameters in response to new information. This capability is a hallmark of true intelligence.
Lacking in Common Sense
Common sense involves understanding things without being explicitly told. It helps us navigate the world effectively. Humans acquire common sense through experiences and interactions with our environment, allowing us to handle new situations and make effective decisions. Without it, we would struggle to navigate unfamiliar scenarios.
The question now follows: how do we encode this common sense in machines? That remains a problem in artificial intelligence today. I don’t know enough yet to answer this question. I do know that common sense in self-driving cars will be crucial for their integration into everyday life, where unpredictable scenarios are common.
Imagine sending your fully autonomous self-driving car to pick up groceries. Despite its advanced capabilities, it encounters a traffic light stuck on red and stops indefinitely. Unlike a human driver, who would quickly assess the situation and find a workaround, the car fails to act because it lacks common sense. This example highlights the importance of developing AI systems that can navigate the complexities of the real world.
Hallucination
Hallucinations occur when a model generates data that is not grounded in truth, especially when such data is presented with high confidence. This issue arises because the modeling process relies heavily on statistical patterns rather than genuine understanding. Consequently, models can learn noise, overfit data, and make errors in encoding and decoding, all of which contribute to hallucinations.
The problem of hallucinations is significant and contributes to the unreliability of large models. For these models to be trusted and adopted in fact-critical tasks, such as research, they need to be more grounded in truth. Developing methods to reduce hallucinations and improve the accuracy of AI outputs is essential for the broader adoption of AI in sensitive and critical applications.
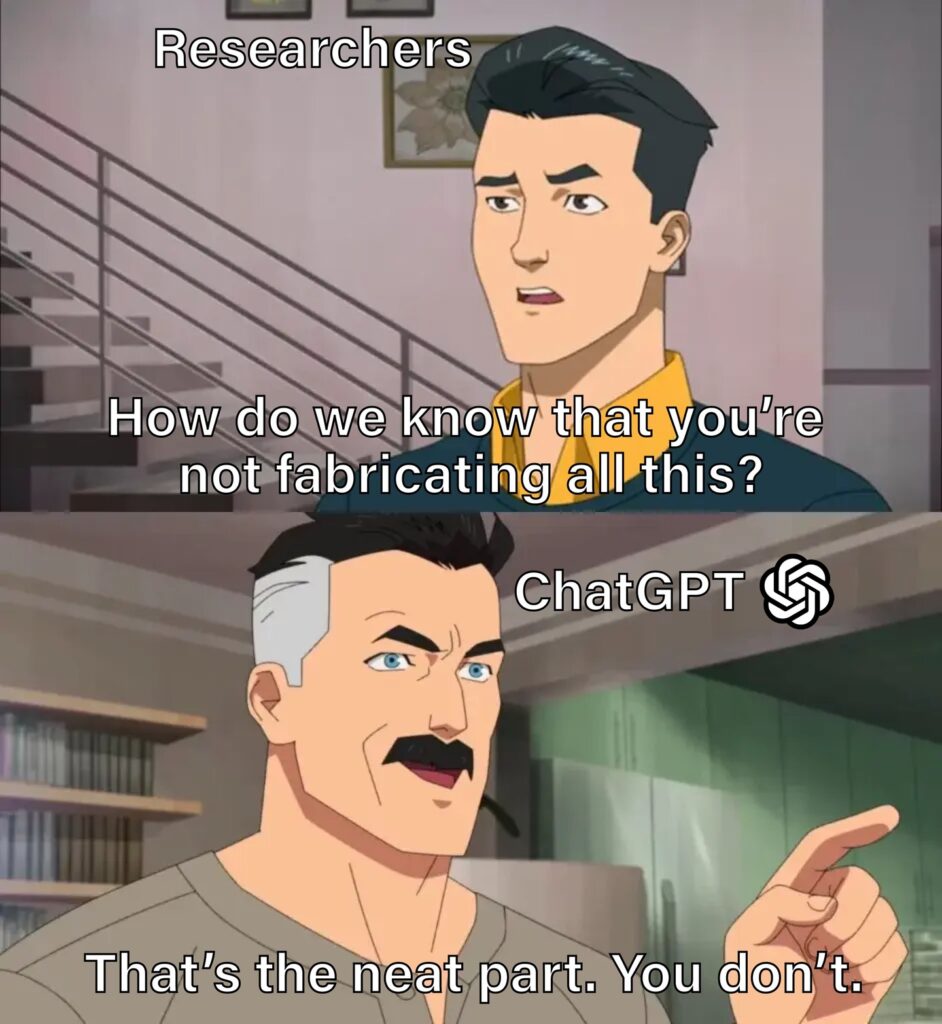
Towards Artificial (General) Intelligence
AGI or Artificial General Intelligence is often viewed as the end goal for AI systems. To put in my own words, AGI is a hypothetical system that can function well, solve problems, and achieve goals across any range of cognitive tasks. There are ongoing debates around the possibility of AGI; and the time frame within which we can expect to achieve it. How will we know when we get there? Will Turing’s Test suffice? Time will tell I guess..
On Scaling laws
Scaling laws suggest that we can enhance AI model performance by increasing certain variables, such as dataset size, number of parameters, and computational infrastructure.
Model performance generally improves with these variables, but at extremely large scales, it starts to plateau, and diminishing returns set in.
Beyond a certain point, adding more computing power, parameters, and data yields only marginal improvements, raising questions about the fundamentals of the underlying approach.
This might explain why Sam Altman claims to need $7 trillion to build AGI. Is the plan to continue relying on brute force?
Surely, leading experts understand that infinite scaling is not a long-term solution. It will take us far though. Although we might be able to scale compute via Quantum or Thermodynamic computing, scaling data will have its limits, even if we synthesize the training data. The long-term solutions lie in addressing the issues and limitations outlined in this note. Good engineering outweighs scaling laws because scaling laws ultimately do not scale.
Ongoing research
Artificial Intelligence is a rapidly evolving field with many exciting advancements on the horizon. This section introduces several concepts and provides relevant links for further reading.
Transfer learning allows models to transfer knowledge from one task to enhance performance on related tasks, reducing the need for retraining from scratch. Techniques like zero-shot, one-shot, and few-shot learning improve data efficiency by training models with few or no examples. Memory Augmented Neural Networks, using meta-learning techniques, enable models to ‘learn how to learn’ and apply previous knowledge to new data. While promising, much work remains.
Improving energy efficiency is a multifaceted problem, requiring advancements in both hardware and software. Techniques like pruning and quantization optimize memory and computation. More energy-efficient model architectures maximize work per unit of energy. Also, improvements in specialized hardware such as GPUs and TPUs can significantly boost energy efficiency.
Chain Of Thought prompting involves using prompt engineering techniques to provide examples of how a model can logically break down a task in order to achieve better reasoning outcomes. COT is powerful but has its limitations. Depending on the quality of examples used in prompting, the model may not perform well on problems requiring nuanced thinking.
For AI systems to reliably handle nuance, they must improve at reasoning. This involves integrating various forms of reasoning into neural network architectures, including causal reasoning (understanding cause and effect), counterfactual reasoning (considering hypotheticals), and abductive reasoning (deducing preconditions from results). Research in this area can draw inspiration from cognitive functions in biological neural networks.
Addressing persistent hallucinations in AI systems is crucial for building trust. Techniques like Retrieval Augmented Generation can help generative models improve the accuracy of their output.
There is ongoing research to incorporate extensible architectures like MRKL into future AI systems. These architectures tend to be neurosymbolic; consisting of neural (intelligent) and symbolic (discrete) elements.

The neural elements are experts which are neural networks that are trained for specialized tasks. Symbolic elements are more discrete, eg a math calculator module, an API, a database, an LDAP server, etc… This architecture allows you to extend your system continuously while adding new experts.
The router is a neural network trained to route tasks to experts based on the input. Output from one expert can serve as input to another expert.
Imagine an MRKL architecture comprising: a weather forecasts expert, Google Calendar API, and Slack API.
Technically, one can issue a prompt like this:
cancel all my meetings next week that fall on rainy days. Also, post an apology message on the team slack channel for the missed appointments.
The router, which should have reasoning capabilities, should be able to break the prompt like this:
- call the Weather expert with question: what are rainy days next week?
- call Calendar API to cancel meetings on days returned in Step 1
- call LLM to generate an apology message for days missed
- call Slack API to publish the message on the team channel.
You get the idea.
One benefit of this architecture is how fallback actions can be delegated to the general-purpose LM, while we continue to extend the system by adding specialized experts and discrete modules.
Countless other problems are being addressed on the research front. If you want to see the latest publishings on Artificial Intelligence, here are a few links:
Closing remarks
AI has undergone several hype cycles; this will not be the first. In the 1980s, AI research hit a significant brick wall due to limited computational power and data availability. However, the gaming industry gave us GPUs which provided specialized hardware for complex parallel calculations. Also, the rise of the internet brought an explosion of user-generated content, providing massive datasets that allowed us to train AI models to mimic intelligence.
As we progress, we are faced with hard facts and constraints. These constraints, however, are beneficial as they spark much-needed creativity and innovation.
Computing will always tend towards simplicity for the user and complexity for the engineer. To make software easy for users, engineers must solve complex problems. The simpler the software, the more complex the problems.
As our understanding of the world expands, so does our ability to manage and leverage complexity. But as we move forward, we must remain bound by ethical constraints. We owe it to ourselves to ensure that AI advancement is guided by principles of fairness, accountability, and transparency.